The Data Fusion
Contest has been organized by the Data Fusion Technical Committee (DFTC) of
the Geoscience and Remote Sensing Society (GRS-S) of the IEEE. In 2007, the
contest was related to urban mapping using radar and optical data. A set of
satellite radar and optical images (ERS amplitude data and Landsat multi-spectral
data) was made available with the task of obtaining a classified map as accurate
as possible relative to ground reference data depicting land cover and land
use classes for the urban area of interest.
The winning algorithm was based on a neural network approach. One of the major
advantages of neural networks with respect to statistically based classifiers
is that NNs draw their own input-output discriminant relations directly from
the data and do not require that a particular form of a probability density
function be assumed. The classification procedure was divided into three steps:
preprocessing, neural network classification and post-processing.
The final classification map, shown in figure, reached the accuracy of 0.9393
in terms of k-coeff. with respect to the unknown ground reference data used
to rank the contest’s results.
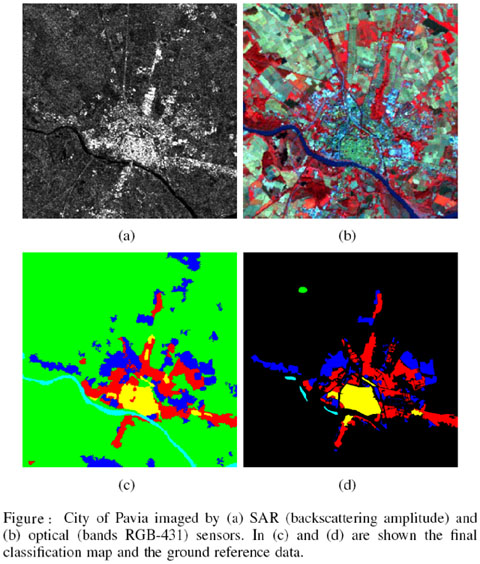
<click
to enlarge>
|